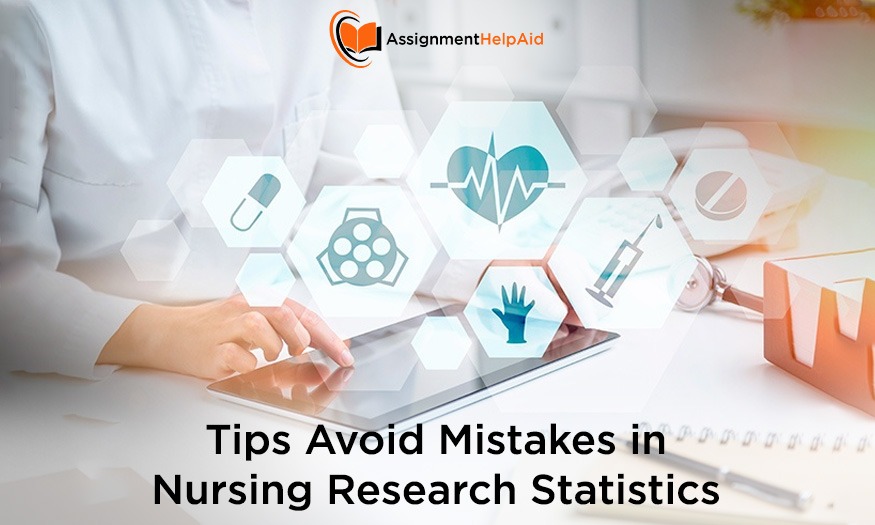
Tips Avoid Mistakes in Nursing Research Statistics
Statistics plays a vital role in nursing because it helps with evidence-based practice, clinical decision-making, and progress in the nursing field. This means that statistical methods are valuable because they improve the quality of care given by nurses, help create healthcare policies, and most importantly, ensure patient safety and positive outcomes.
Role in Evidence-Based Practice: In evidence-based practice (EBP), statistics helps analyze research data. This helps nurses figure out which interventions work best, support clinical recommendations, and plan care using methods like meta-analysis and systematic reviews.
Clinical Decision-Making: Statistics provides valuable insights into clinical practice, helping nurses make informed decisions about patient prognosis and treatment effectiveness. This improves patient safety by ensuring the best possible care.
Advancing the Nursing Profession: Statistics is crucial for nursing research and improving healthcare quality. Analyzing healthcare data helps find reliable outcomes to enhance healthcare systems.
Sometimes, nursing students find statistics challenging because they lack an understanding of basic concepts. That’s why many students seek nursing statistics assignment help during their coursework. It’s important to avoid statistical errors and use correct methods to ensure accurate results and patient safety.
Common Mistakes in Nursing Research Statistics: Assignment Help
Mistake 1: Choosing the Wrong Statistical Test
Explanation: Statistical tests help find important results for a research question. However, using the wrong test can lead to an invalid analysis. This happens when the test doesn’t match the type of data collected or the research goals.
Example: For example, using a t-test to compare means when the data is categorical, like different types of wound dressings, can give false findings.
How to Avoid: To prevent this, it’s helpful to consult with a statistician. They can make sure the research questions, hypotheses, and statistical methods are a good match. This leads to better decisions in selecting the right study design and tests and getting accurate and valid results.
Mistake 2: Ignoring Sample Size Importance
Explanation: Small sample sizes mean data might not show significant differences or connections. Even if there’s a real effect, a small sample might miss it, leading to false results or null hypotheses.
Example: For instance, a study with only 10 patients might not show if a new pain management method works well because of the small sample size. This could give unreliable results.
How to Avoid: To fix this, it’s important to do a power analysis early in the study. This helps estimate the minimum number of samples needed for accurate findings. Establishing enough power for a study prevents these mistakes and ensures true effects can be found, making the findings more credible.
Mistake 3: Mixing Up Correlation and Cause
Explanation: A common misunderstanding is thinking that if two things are linked, one must cause the other. Correlation means there’s a connection between two things, but it doesn’t show that one thing makes the other happen.
Example: For example, there might be a link between drinking coffee and fewer cases of Alzheimer’s disease. But that doesn’t mean coffee prevents Alzheimer’s. Other factors could be involved, like people who drink more coffee also having healthier lifestyles.
How to Avoid: To tell if one thing causes another, researchers should do experiments. In an experiment, they change one thing and see how it affects something else. This helps figure out if there’s a real cause-and-effect relationship.
Mistake 4: Missing or Incomplete Data
Explanation: Not having data for everyone can make results and conclusions unfair. Sometimes data might be missing or incomplete9, which can lead to wrong results.
Example: If a study about patient falls doesn’t have info about their health before falling, it’s hard to know what causes falls.
How to Avoid: To fix this, collecting and checking data properly is important. Using the right statistical methods, like filling in missing data or finding averages, can help make results fairer.
Mistake 5: Dealing with Outliers
Explanation: Strange data points, called outliers, can mess up results and lead to wrong conclusions, especially when finding averages.
Example: In a study about blood pressure, one person with very high blood pressure because of another health problem could make the average look too high.
How to Avoid: Researchers should know how to find and handle outliers. They can use different methods to deal with outliers, like checking if they’re wrong or changing how the data is shown. Deciding what to do with outliers depends on what the study is about.
Mistake 6: Reading Too Much into Statistical Results
Explanation: It’s important to understand that just because something is statistically significant doesn’t always mean it’s important in real life. There’s a difference between what’s statistically significant and what’s meaningful.
Example: For instance, a study might show that one treatment reduces pain more than another treatment, but the difference might be so small that patients wouldn’t notice.
How to Avoid: Students should learn to look at more than just p-values. They should also consider things like effect size and confidence intervals. Effect size tells you how big the difference is between groups, while confidence intervals give a range of values where the true effect might be. This helps make sure that findings from a study are both statistically and clinically meaningful.
Mistake 7: Using Misleading Graphics
Explanation: Badly made graphs or charts can make data look different from what it is. This can confuse people who look at the data.
Example: A bar chart that changes the scale on the y-axis to make differences look bigger than they are could be misleading. If the scale is cut off, differences seem bigger than they are.
How to Avoid: Make sure to follow guidelines for making clear and honest data visuals. Transparency in how you show your data helps keep your work trustworthy.
Mistake 8: Ignoring Ethical Concerns
Explanation: Being ethical means doing the right thing, especially in research. This includes things like protecting people’s privacy, not changing data, and showing all your results.
Example: Only showing the most exciting results from a study is unfair because it doesn’t give people the full story.
How to Avoid: Researchers need to stick to ethical rules when they do research and share their results. This means being clear about how you did your research and showing all the information you find, even if it’s not as exciting. Being ethical is important for making sure research is valid and puts patients first.
Conclusion
Statistics is essential in nursing research, aiding evidence-based practice and clinical decision-making. However, common mistakes can hinder accurate analysis. To avoid these, nurses should choose appropriate tests, ensure adequate sample sizes, understand correlation vs. causation, collect complete data, handle outliers effectively, interpret significance correctly, use clear visualisations, and adhere to ethical principles. Nurses can enhance research quality and improve patient outcomes by addressing these pitfalls.
Nursing Assignment Help for Statistics and Research
Need help with nursing assignments involving research and statistics? You’ve come to the right place. With over 20 nursing specialists and biostatisticians who have a ton of experience in nursing, healthcare, and biostatistics, we’re here to offer comprehensive assistance with nursing statistics assignments. Our expertise covers everything from basic descriptive statistics to advanced analysis techniques like correlation, regression, and survival analysis. Here are some key features of our services:
- We use standard software tools like SPSS, SAS, R, and Stata to provide accurate data analysis solutions, and we include detailed reports for each section of the assignment.
- Our team ensures that the right statistical methods are applied according to your instructions and the data provided, so you can trust the accuracy of the results.
- We interpret all analyses clearly and precisely, presenting the results in tables, diagrams, plots, and charts for easy understanding.
- We believe in transparency, so we include all results in our reports, even if they’re not essential, to ensure credibility and data awareness.
- We aim to help you build a strong foundation in nursing statistics, assist with data analysis, and support you with your assignments through our nursing homework help services.